Extract, transform and load pipes that understand language
ETL pipelines are the unsung heros that enable your business to make complex analysis of business relevant data. Why? Because generally, data is a mess. If you have multiple sources, you need to first standardize the schema and then store transformed data in a centralized space.
Until now, your ETL pipelines are missing out a crucial thing: insights from textual data!
With our platform, building ETL pipelines that can also standardize and categorize insights from textual data is super simple. Let's dive into one example.
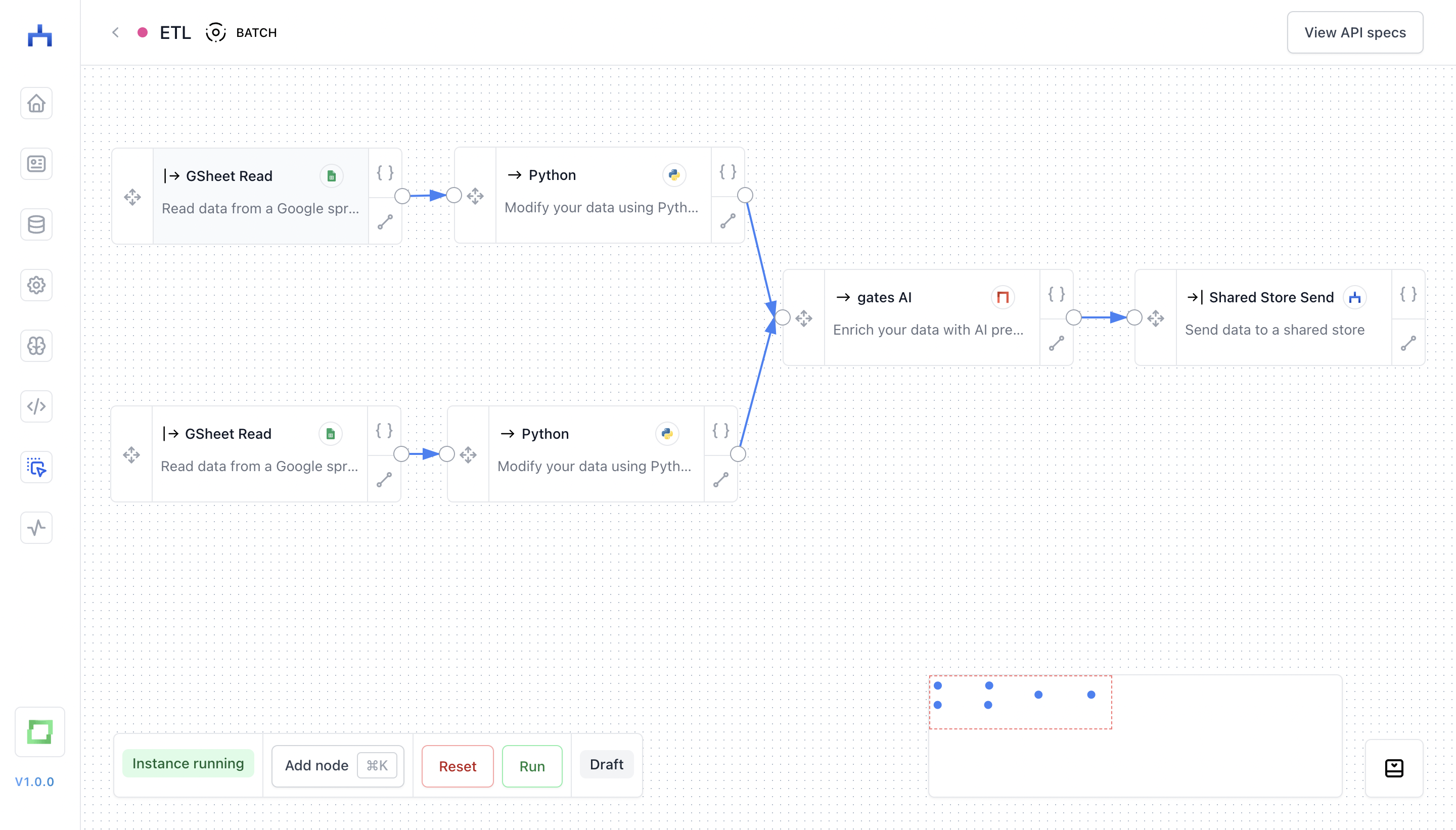
Let's say that we have two Google spreadsheets containing data from webforms or something similar (of course, this could be any other data source). An ETL pipeline could look as follows:
- for every data source, we have a simple Python script standardizing the very structure, e.g. renaming columns from
"headline"
to"text"
or similar; - the standardized data schema is fed into a gates node, which in turn enriches your unstructured texts with structured insights (e.g. sentiment, intents and such);
- the transformed data is put into a shared store, which can be fetched via API.
You see, it's quite straight-forward. And with the capabilities of workflow, you can build both simple or highly-complex ETL pipelines!